What is Generative AI
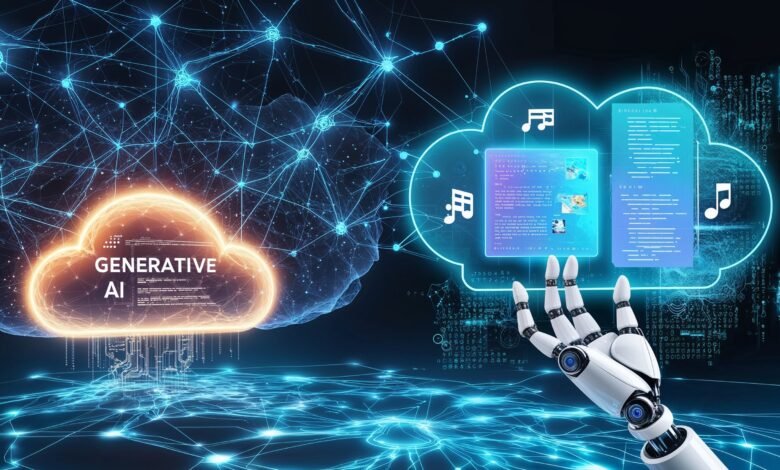
What is Generative AI?
Discover what is generative AI, how it works, its applications, benefits, limitations, and future potential. Explore the creative side of artificial intelligence.
Introduction:
Generative AI refers to algorithms that are designed to create new content or data that mimics existing content. Unlike traditional AI, which typically classifies or processes data, generative AI produces something entirely new. This includes generating text, images, music, and even complex programs, all without human intervention. The most famous examples of generative AI today include models like OpenAI’s GPT-3, which can write human-like text, and DALL-E, which generates highly detailed images from text prompts.
How Does Generative AI Work?
Generative AI primarily relies on two key technologies: neural networks and deep learning. These systems are trained on massive datasets to understand patterns and relationships in data. After training, the AI model can create new outputs that follow these learned patterns.
- Neural Networks and Deep Learning
Neural networks are the building blocks of generative AI, mimicking the structure of the human brain to process data. Deep learning, a subset of machine learning, uses multiple layers of these neural networks to interpret large amounts of complex data. - Training with Large Datasets
Generative AI models require large datasets to learn from, such as collections of text, images, or sounds. These datasets enable the models to generate new outputs that are convincing and useful. - The Role of Models Like GPT and DALL-E
Pre-trained models such as GPT-3, GPT-4, and DALL-E have gained significant attention for their ability to generate high-quality text and images. These models use a vast number of parameters to generate creative outputs from a given prompt.
Types of Generative AI:
- Generative Adversarial Networks (GANs)
GANs are made up of two networks: the generator and the discriminator. The generator creates content, while the discriminator evaluates it. This back-and-forth results in increasingly realistic outputs. - Variationally Autoencoders (VAEs)
VAEs are models that learn to encode data into a simplified representation and then decode it into a new form. This process is used for applications like image generation. - Transformers (e.g., GPT-3, GPT-4)
Transformers are models designed for sequential data, such as text. They generate new sequences (like sentences or paragraphs) based on what they have learned from previous examples.
Applications of Generative AI:
Generative AI has a wide range of applications across various industries:
- Text Generation (e.g., Chatbots, Content Creation)
Generative AI powers chatbots, automated writing assistants, and even journalism tools that create articles and reports. - Image Generation (e.g., Art, Design)
Tools like DALL-E and MidJourney allow users to generate detailed artwork and designs based on simple text descriptions. - Video and Audio Creation
Generative AI is being used to create deepfake videos, as well as generate lifelike music and voiceovers. - Code and Software Development
AI models like GitHub’s Copilot assist developers by generating code snippets, automating repetitive tasks, and improving overall productivity. - Game Design and Simulations
Game developers use generative AI to create characters, landscapes, and even entire game narratives dynamically.
Benefits of Generative AI:
Generative AI has numerous benefits, including:
- Automation and Productivity:
Automates creative tasks that would take humans much longer to complete. - Creative Assistance and Innovation:
Helps artists, writers, and designers by generating new ideas. - Personalization and Customization:
Creates highly personalized content for users in marketing, entertainment, and more. - Rapid Prototyping:
Enables companies to quickly generate new product designs or software prototypes.
Challenges and Limitations of Generative AI:
Despite its many benefits, generative AI faces several challenges:
- Ethical Concerns
Deepfake technology and AI-generated content raise questions about authenticity and manipulation. - Data Privacy Issues
Generative AI models require access to large datasets, often involving sensitive or private information. - Bias in AI Outputs
If trained on biased data, generative AI models can reproduce and even amplify these biases. - Energy Consumption
Training large generative models is energy-intensive, raising concerns about environmental impact.
Generative AI vs. Traditional AI:
- Key Differences in Functionality
Traditional AI typically classifies or predicts data, while generative AI creates new content. - When to Use Generative AI vs. Traditional AI
Generative AI is best suited for creative tasks, while traditional AI is ideal for analysis and classification.
Generative AI and Ethics:
Ethical considerations surrounding generative AI are critical, particularly in areas like copyright and misinformation.
- Copyright Issues
Generative AI often uses copyrighted material for training, leading to legal concerns. - Deepfakes and Misinformation
The ability to generate lifelike fake videos and images can be misused for malicious purposes. - Accountability and Transparency
Who is responsible for the content created by AI? This is a pressing ethical question with no easy answers.
How to Implement Generative AI in Business:
- Choosing the Right AI Tools:
Selecting the right platform and tools depends on the specific needs of the business, whether for text, image, or video generation. - Integrating Generative AI with Existing Systems:
Businesses can integrate generative AI to streamline workflows, enhance creativity, and automate repetitive tasks. - Case Studies of Successful Implementations:
Many companies, including Netflix, IBM, and Adobe, have successfully implemented generative AI to improve customer engagement and productivity.
The Future of Generative AI:
Conclusion:
Generative AI is revolutionizing the way we create content, from text to images to music. While it offers immense benefits in terms of automation and creativity, it also presents ethical challenges. As we move into the future, the potential of generative AI will continue to expand, making it an essential tool in various industries.
FAQs
- What is generative AI used for?
Generative AI is used for creating text, images, videos, music, and more. It is applied in industries ranging from marketing to entertainment. - How is generative AI different from traditional AI?
While traditional AI focuses on analyzing and classifying data, generative AI creates new content based on existing data patterns. - Is generative AI ethical?
Generative AI raises ethical concerns, including data privacy, bias, and the potential misuse of deepfake technology. - What are GANs?
GANs (Generative Adversarial Networks) are a type of AI that generates new data through a competition between two networks: the generator and the discriminator. - Can generative AI be used in business?
Yes, businesses use generative AI for various purposes, including content creation, marketing personalization, and product design.